Traceability for trusted multi-scale data and fight against information leak in artificial intelligence systems in healthcare
Coordinating Partners : Gouenou Coatrieux
Coordinating institution : Inserm – Regional Delegation Grand Ouest
Traceability, trusted medical data, data governance, fight against information leaks, trusted artificial intelligence, provenance, consent management, cryptography, cryptowatermarking
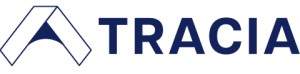
In healthcare, cybersecurity is at the heart of the challenges of artificial intelligence (AI) empowered by massive multiscale data and new medical practices. It is also imposed by means of a complex broad set of strict ethic and legislative regulations. On one hand, the security of data should be ensured whatever their nature, transmission, processing and transformations they went through.
On the other hand, methods that will be applied to exploit these data must themselves be safe. Healthcare AI systems are considered as of high risk by EU.
In this context, among security objectives traceability becomes more and more critical, especially with the externalization of data and of their processing. On one side, there is a need to be sure of the origin of the data, of their history, how they have been created, processed, and so on, to enable a trustworthy and secure development of AI. The same questions arise regarding machine learning (ML) models built over these data. One need to know how they were created because of their use in daily practice. On another side, it is also necessary to empower patients to act on their personal health records giving them the capability to manage their consent. Health professionals have similar concerns. At least, there is a need to fight against information leak or disclosure (more than 55% of the attacks come from the inside). As defined, traceability encompasses different challenges at the frontier between cybersecurity, data management and processing according to patient and health professional agreement, that should be simultaneously addressed while considering existing standards and practices.
TracIA aims to meet these traceability challenges as a whole at the scale of a national learning medical information system (LMIS). LMIS stands on the massive reuse of data to extract new knowledge to serve new systems, next deployed in LMIS for medical practice and producing new data that LMIS can reuse to create new knowledge. LMIS can easily support the PEPR DH goal to design a patient digital twin. Concretely, TracIA proposes to develop efficient cutting edge traceability methodologies and technological means; the missing building blocks required to develop reliable and pioneering AI based systems in healthcare; to reach several objectives at once:
- Data governance and patient consent management in time – In an effort to comply with national and international regulations (e.g. GDPR, e-IDAS), TracIA aims at achieving data governance at the scale of LMIS, beyond data-warehouse governance, considering that patients and health professionals want to manage their own consent policy. These are key challenges to address.
- Management and certification of low-level (fine-grained) data provenance – To enforce the trustworthiness of the use and reuse of data, one should be able to retrace the history of a piece of data/ML models, in terms of transmission, production, manipulation/transformation but not only. This is a core issue regarding the certification of AI based decision aid systems in healthcare. Scientifically, it is also critical in terms of reproducibility. There is a need for innovative technical means to trace/ retrieve patient data, while preserving privacy.
- Fight against information leaks – Preventing, identifying and remediating information leaks are crucial questions in healthcare that are still without concrete responses especially in the context of LMIS where data are massively externalized, distributed and shared. For this TracIA will develop innovative crypto-watermarking and AI based data leak prevention solutions.
To reach these objectives, TracIA brings together a multidisciplinary consortium of experienced researchers with expertise in cybersecurity, computer and data sciences, medical informatics, information processing and human and social sciences with privileged access to technical platforms to evaluate and validate TracIA solutions.
Laboratory or department, team | Supervisors |
LaTIM – U 1101 – Eq Cyber Health | Inserm, Bretagne Occidentale University, IMT Atlantique
CHRU Brest partner |
LTSI – U 1099 – Eq DOMASIA | Inserm, Rennes University
CHU Rennes et CNRS partners |
SAMOVAR – UMR 5157 | CNRS, Mines Telecom, Telecom Sud Paris, IP Paris |
Lasco Idea Lab | IMT Atlantique |
LIFO – EA 4022 | INSA Centre-Val de Loire, Orléans University |
LIST | CEA, Paris-Saclay University |